The State of the Art in Intrusion Prevention and Detection Source Quality
Abstract
The internet connected devices are prone to cyber threats. Near of the companies are developing devices with congenital-in cyber threat protection mechanism or recommending prevention measure. But cyber threat is condign harder to trace due to the availability of various tools and techniques to bypass the normal prevention measures. A data mining-based intrusion detection system can play a key function to handle such cyberattacks. This newspaper proposes a threefold arroyo to analyzing intrusion detection system. In the first phase, experiments have been conducted by applying SVM, Determination Tree, and KNN. In the second phase, Random Forest, and XGBoost are applied every bit lately they accept been showing significant improved performance in supervised learning. Finally, deep learning techniques, namely, Feed Forrad, LSTM, and Gated Recurrent Unit neural network are applied to conduct the experiment. Kyoto Honeypot Dataset is used for experimental purpose. The results show a meaning comeback in IDS outperforming the state of the arts on this dataset. Such improvement strengthens the applicability proposed model in IDS.
Introduction
The rapid development of computational and intelligent devices, smart dwelling house appliances, and high-speed internet enables everything to be continued. Educational institutions, business concern organizations, industries are becoming heavily dependent on technological devices. Equally a upshot, there is a tremendous risk of cyberattacks. An effective measure is essential to defend against such attacks. Furthermore, at that place is a lot of work with different policies to defend threat [one] such as software developments with quality of services, and parallel technologies in Cisco Switches [2], and intrusion detection in calculator networks with big information lightweight model [three].
In recent years, data science technique has been adapted to implement effective Intrusion Detection System (IDS). Supervised learning algorithm tin help to solve the nomenclature problem of network intrusion detection [iv]. There are numerous implementations of machine learning and deep learning-based IDS. Several algorithms have been used to develop IDS, such as Naïve Bayes, Cocky Organizing Map (SOM) [v], non-dominated genetic algorithm [6], Support Vector Machine (SVM) with Softmax or radial footing function (rbf). Each of these models is different, just their goal is to differentiate the normal traffic from the compromised ones.
Deep learning, on the other hand, has been applied in a broad variety of applications, including sound signal processing [vii], image processing [8], speech processing [9], etc. In [x], Kohonen self-organizing map has been applied to detect network intrusion. The authors emphasized that it can automatically categorize the varieties of input during training, so it can fit the new data input to evaluate the operation. A case report on IDS can exist found in the literature where authors used rbf Neural Network (NN) [11].
Feature option is used to excerpt relevant features that describe the original dataset precisely. It enhances the model performance [12] through the emptying of inessential or noisy features [13] without baloney of original data pattern and without removing essential features. The removal process can exist performed manually or with the help of algorithms like univariate feature selection [14], correlation-based characteristic choice [15], minimum redundancy maximum relevance algorithm (MRMR) [xvi].
To analyze IDS, this paper selects Kyoto Academy Honeypot dataset [17] for experimental purposes. Although several experiments are conducted using this dataset, very few of them are based on Long Short-Term (LSTM) Recurrent Neural Network (RNN). Even at that place is no experiment with boosting technique establish in the literature. This paper adopts an approach to combine the auto learning model with several deep learning techniques. After collecting the dataset, a set of preprocessing method is applied to clean the dataset. A subset of features is then selected using a suitable feature option technique. Various feature selection techniques are applied to understand their effect. Afterwards selecting the features, the dataset is balanced and fed to dissimilar classifiers to train the model to perform the prediction. The ensemble learning algorithm is implemented forth with optimization in Boosting-based classifier. A detailed comparison is made with state-of-the-art to evaluate the strength of the proposed model. In improver, an explainable tool is also applied hither equally it interprets how the model is making decisions and predictions and executing actions [eighteen].
The balance of the paper is organized as follows. Section 2 critically analyzes state-of-the-art. An overview of the dataset is given in Sect. 3 along with its exploratory analysis. Section 4 presents the overall methodology proposed in this newspaper and portrays the steps adopted in the experiments. After illustrating the experimental setup in Sect. five, the obtained results are analyzed, and comparative analysis with the state-of-the-art are performed in Sect. vi. Finally, Sect. 7 summarizes the paper forth with outlining the strength and weaknesses of the proposed arroyo.
Literature Review
The authors in [nineteen] experimented on Kyoto 2006+ dataset to build IDS. The authors implemented SVM, Naïve Bayes, Fuzzy C-Means, Radial Footing Function, and ensemble methods. The SVM, KNN, and Ensemble Method accuracies were identified as 94.26, 97.54, and 96.72%, respectively.
The author of [20] proposed GRU SVM and GRU Softmax IDS models on Kyoto 2013 dataset. Softmax activation role works meliorate with multiclass nomenclature. Therefore, GRU SVM outperformed GRU Softmax for binary classification, because the accuracies as 84.15 and 70.75%, respectively.
Vinayamkumar et al. [21] proposed a deep neural network model, justified the model with diverse intrusion detection dataset, including Kyoto 2015 dataset. The author developed NN with v layers. The NN accuracy and recall for intrusion detection are 88.v%, with an excellent recall value of 0.964. The author provided a comparative study on KNN, SVM rbf, Random Wood, and Determination Tree, where accuracies and recalls were noted as 85.61 and xc.5%, 89.5 and 99.5%, 88.2 and 96.3%, 88.three and 88.3%, respectively.
Javaid et al. [22], developed deep learning based cocky-taught learning for intrusion detection. The authors implemented a sparse autoencoder with softmax activation function, and STL accuracy is 98% in NSL KDD Dataset.
Authors of [4] developed an intrusion detection system by applying four supervised algorithms. The authors generated 75% accuracy and 79% recall in SVM. The model gained 99% accuracy and call up in Random Forest Classifier.
Almseidin et al. [23] adult IDS with Random Forest and Determination Tree and listed accuracies as 93.77 and 92.44%, respectively, on KDD Dataset.
Costa et al. [24] performed nature inspired computing with optimum path wood clustering, SOM, and Grand-means for developing effective IDS. The authors used KDD Cup and NSL KDD Loving cup dataset along with more than 6 datasets for their experimentation and considered purity measures for evaluation metric.
Ring et al. [12], provided a comparative written report on the bachelor dataset to build IDS. AWID focused on 802.11 networks, CICIDS2017, DDoS2016, KDD Loving cup 99, Kent-2016, NSL KDD, SSHCurve and many other dataset repositories. The authors considered some parameters to evaluate each dataset. The parameters are general data, nature of data (format, meta, anonymity), record environment (network organization or honeypot organisation, etc.), evaluation based on predefined split, balance imbalance, labeled or not. In the case of Kyoto Dataset, it is labeled imbalanced, with no predefined splits from real traffic of honeypot organization.
Dataset Overview
A brief exploratory profile of the Kyoto Academy Honeypot System 2013 dataset has been discussed in this section.
Backdrop of Kyoto Dataset
The belongings of Kyoto Dataset is described in Table 1. The publicly available dataset comprises both flow-based and parcel-based data, which contains normal and attack traffic. Therefore, the nature of the data is mixed blazon [12]. The dataset is generated from real honeypot traffic and excludes the usage of Servers, Routers, BoTs.
The experimental dataset size is 1.99 GB zipped file. A few days are missing among 365 days of 2013. Information technology stores a continuous fourth dimension data stream. It has 24 features, among which ten features are taken from the KDD dataset, and 14 features are created by the authors of [25].
Exploratory Data Assay (EDA)
In our dataset, amidst 24 features, xv features are continuous and quasi continuous, and nine features are categorical. In the preprocessing part, we have performed the necessary computation to requite a perfect shape to the information.
As shown in EDA Table 2, later on preprocessing, there are 11 numeric, 8 Boolean features, and three features marked as either highly correlated or constant.
Features Exploration
The post-obit graphs, created with pandas_profiling, present important insights. The graphs are also elucidated to sympathise the shape and format of information to gain the best insight.
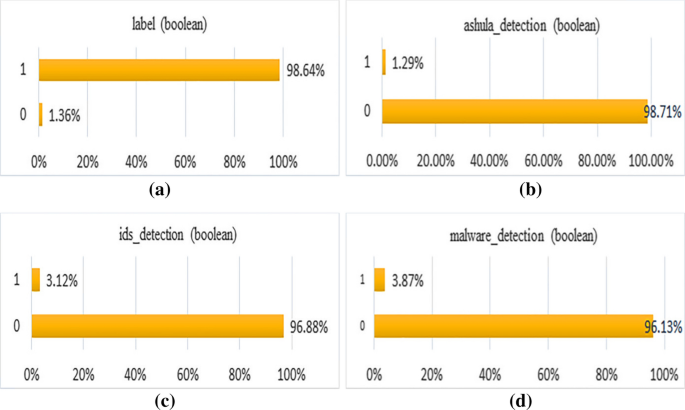
Bar chart of some features
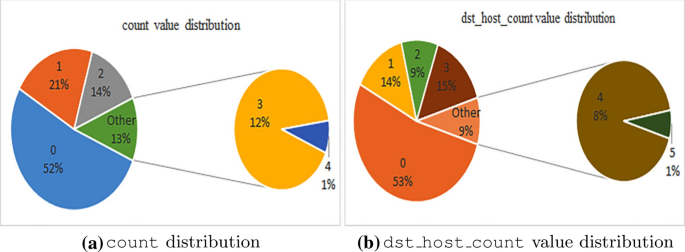
Features with pie charts
- 1.
label: It is to mention 0 = Normal, 1 = Assault for label. Every bit shown in Fig. 1a, nosotros observe that 98.64% is Attack. Hence class imbalance insight is observed since merely i.36% of total instances belong to the Normal grade.
- 2.
ashula_detection: Whether shellcodes and exploit codes were used in the connection, '0' = used at 98.71% rate, and 'ane' = non used at 1.29% charge per unit as shown in Fig. 1b.
- three.
ids_detection: In Fig. 1c, 0 = no alert triggered 96.88% times, and ane = alert triggered 3.12% for ids detection.
- 4.
malware_detecion: In Fig. 1d, 0= malicious software not observed at 96.13%, 1= malicious software observed at 3.87%.
- five.
Features with like distributions: The count characteristic in Fig. 2a contains values where 52% are 0. And, the dst_host_count as well contains distribution of feature in Fig. 2b, having 53% of pie nautical chart are 0.
Similar types chart with different value counts has been observed in our dataset for rest of the features. A detailed description of all features can be found in [17].
Proposed Methodological Framework
A serial of steps take been taken, from dataset cleaning, characteristic selection, dataset balancing to perform nomenclature, in this work. Figure iii illustrates the whole workflow of the proposed approach where each footstep of the workflow is described in the post-obit sections.
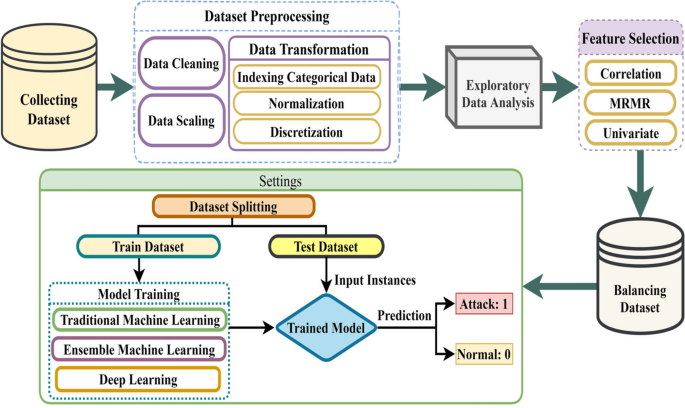
Schematic diagram of the workflow
Information Preprocessing
A dataset containing dissonance, null values, and irregular shape would atomic number 82 to the fatal performance of model [26]. In that location is a list of mandatory processes to format the dataset accordingly to overcome and avoid catastrophic performance. As shown in Fig. 3, the procedures are (i) Data Cleaning, (two) Data Scaling, and (3) Data Transformation.
Information Cleaning
The experimental dataset instances are free of NAN values. But there were duplicate instances that were dropped from the dataset. According to the dataset, ids_detection, ashula_detection, and malware_detection contain string and numerical value. Cord values are assigned integer 'i' to rescale features. Otherwise, information technology is kept as '0'. For instance, the label contains 3 types of values, i.e., '1'= Normal, '\(-1\)' and '\(-ii\)' = Attack. It is required to rescale '\(-ane\)' and '\(-2\)' by replacing with '1' (Set on) and rescaling the 'i' with '0' (Normal). So now, the label is '0' and '1', indicating 'Normal' and 'Attack' class, respectively. The start_time characteristic is a time frame data, then it is also scaled to continuous data.
Chiselled Information Label Encoding
Well-nigh machine learning and deep learning algorithms accept numerical inputs. That is why categorical data requires to be encoded to numerical inputs to build an efficient model. Label Encoding is a popular encoding technique for converting categorical variables into continuous variables. Label Encoder replaces categories from 0 to n-1 (in terms of our work), where n is the number of variable's distinct categories, numbers for these cardinalities are assigned arbitrarily. The python sklearn library has a class preprocessing which contains LabelEncoder(). The dataset [17] has nine categorical features. The categorical features are transformed to numerical features through applying LabelEncoder(). Normalization is applied later indexing the chiselled data.
Normalization
The raw values of features have a degradation impact on Machine Learning and Deep Learning algorithms. Therefore, normalization is essential to transform the values into scaled values which increase model efficiency as well.
Standardization: In this experiment, first standardization of the dataset is applied to transform continuous and quasi continuous attributes. Python sklearn library has preprocessing grade which contains StandardScaler() to convert continuous and quasi continuous features to the continuous features. Standardization can be denoted as \(z = \frac{ten-\mu }{\sigma }\), where z = generated value, \(\mu\) = mean, and \(\sigma\) = standard deviation.
Min–Max Normalization: The Min–Max normalization is used to transform information into a range of 0–1. Since minimum and maximum values of features are unknown and the dataset is unbalanced, the process is applied before dataset splitting and after balancing to prevent the biasness acquired by outliers of imbalance dataset [26]. The Min–Max normalization can be described mathematically as \(x_{{\mathrm{new}}} = \frac{ten - x_{{\mathrm{min}}}}{x_{{\mathrm{max}}} - x_{{\mathrm{min}}}}\), where \(x_{{\mathrm{new}}}\) is transformed value.
Discretization
Non-standard probability distributions, similar skewed distribution, exponential distribution, shrink model performance. Binning is one kind of discretization method that tin can transform data into a discrete probability distribution. Thus, each numerical value is assigned a label maintaining an ordered relationship which will raise the model performance. Pandas qcut() binning is used for our piece of work, where it returned labeled minimum and maximum values.
Feature Pick
The src_ip_add and dest_ip_add lack significant insights, since these were processed multiple times to hide the bodily addresses due to security concerns [17]. And so, these features were removed, keeping 22 features. Furthermore, the pandas_profiling detects two constant features which are also removed from the dataset. The dataset has twenty features, among which label is the target feature. We have performed a threefold feature selection arroyo to find which algorithm is providing the best outcome.
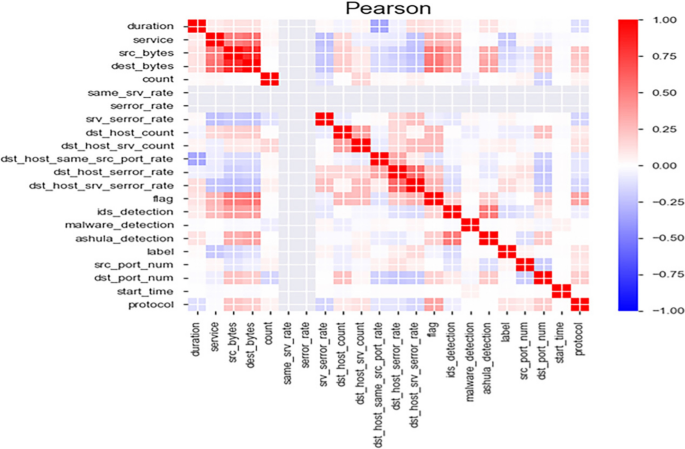
Pearson's correlation matrix of features from author's work [27]
Correlation, Univariate, and MRMR methods are initially applied for characteristic selection. In terms of Univariate Feature Option and MRMR algorithm, there is just i feature deviation compared to Correlation Feature Selection. In correlation matrix dest_bytes is removed, whereas MRMR removed a feature named as dst_host_same_src_port_rate. Univariate feature selection as well does a similar blazon of ciphering. Since there is no significant divergence amidst the three methods, the features are selected based on the correlation matrix.
In general, features with lower dependency measures are considered independent features. Pearson Correlation coefficient (\(\rho\)) [28] measures the degree of linear correlation between two variables. In our instance, 2 features with \(\rho \ge 0.seven\) will be considered equally highly dependent features. Therefore, observing from Fig. 4 that dest_bytes is highly correlated with src_bytes where \(\rho =0.8253\). Hence, one of the ii features is dropped. Finally, 19 features are selected for the experiment.
Neural Network Layers
Neural Network has enabled the development of intelligent machines that can learn from the patterns and propose accordingly. In the post-obit sections, different neural network layers are introduced, which are implemented every bit the proposed IDS model using Keras.
Dumbo Layer
The dense layer is a non-linear regular deeply continued layer. The more layers are added to the neural network, the more information technology will be complex. Thus, it is chosen the deep neural layer due to the increment of computational complexity with the depth of layers.
Dropout Layer
Dropout layer controls overfitting problem of neural network past preventing co adaptations amid nodes of the layers while training the model [29, thirty]. How much co adaptations would be controlled is usually defined within the range of 0 and 1. In our instance, the dropout parameter is set equally twenty%.
Batch Normalization Layer
Nosotros used this layer while modeling the LSTM and GRU neural network. Neural network model training is a circuitous task due to changes in distributions of data in each layer. The layer performs normalization to make the network stable. In this way, normalizing the inputs with zero mean and unit standard deviation, the neural network trains and predicts faster [31].
Feed-Frontwards Neural Network
In our model, we have used five layers, namely an input layer \(x \in {\mathrm{I\!R}}^d\), \(d \in {\mathbb {North}}\), three subconscious layers (h), and ane output layer (y) with single node which will provide either 1 or 0 for a vector input. Now, below mathematically observing how it works [32].
Input layer or first layer takes \(x \in {\mathrm{I\!R}}^d\), \(d \in {\mathbb {N}}\) equally input. Then, layer passes the information to the next layer, which is offset hidden layer \(h^ane\) denoted as follows:
$$\begin{aligned} h_i^{(1)}\,=\, & {} \varphi ^{(i)}(\Sigma _j\omega _{i,j}^{(one)}x_j+b_i^{(1)}), \end{aligned}$$
(ane)
$$\begin{aligned} h_i^{(two)}\,=\, & {} \varphi ^{(2)}(\Sigma _j\omega _{i,j}^{(2)}x_j+b_i^{(2)}), \end{aligned}$$
(ii)
$$\brainstorm{aligned} h_i^{(iii)}\,=\, & {} \varphi ^{(3)}(\Sigma _j\omega _{i,j}^{(three)}x_j+b_i^{(3)}), \terminate{aligned}$$
(3)
$$\begin{aligned} y_i\,=\, & {} \varphi ^{(4)}(\Sigma _j\omega _{i,j}^{(4)}h_j^{(3)}+b_i^{(iv)}), \end{aligned}$$
(4)
where activation part \(\varphi :{\mathrm{I\!R}}\rightarrow {\mathrm{I\!R}}\), \(b=\) bias, \(\omega =\) directed weight, i node number, and superscripts are the layer number, and j is node number of the previous layer. The \(\omega _{i,j}^{(l)} h_j^{(fifty-ane)}\) indicates that the weight of the current layer is getting multiplied by the output of the previous layer. A relevant FFNN IDS model is proposed in the experimental setup section.
Long Short-Term Memory (LSTM)
Recurrent seems like a recursive part which calls itself often. Recurrent in the concept of a neural network is a cyclic approach that implies the aforementioned computation is repeated recursively on each element of the dataset. RNN is prone to vanishing and exploding gradient issues. LSTM and GRU are ii variant of RNN which solve RNN bug [33]. LSTM contains Input Gate (I), Output Gate (O), and Forget Gate (F). The functionality of these gates of LSTM cell can exist denoted mathematically as follows:
$$\begin{aligned} I_t\,=\, & {} \sigma (Due west^ix_t+U^iH_{t-1}), \end{aligned}$$
(five)
$$\begin{aligned} F_t\,=\, & {} \sigma (West^Fx_t+U^FH_{t-1}), \stop{aligned}$$
(6)
$$\begin{aligned} O_t\,=\, & {} \sigma (W^Ox_t+U^OH_{t-1}), \end{aligned}$$
(seven)
$$\begin{aligned} C'_t\,=\, & {} \sigma (W^{C'}x_t+U^{C'}H_{t-ane}), \end{aligned}$$
(8)
$$\begin{aligned} C_t\,=\, & {} I_t *C'_t + F_t *C'_{t-1}, \terminate{aligned}$$
(9)
$$\brainstorm{aligned} H_t\,=\, & {} O_t *\tanh (C_t). \stop{aligned}$$
(ten)
Here Westward, U are weight matrices. Co-ordinate to the author [34] of LSTM, the equations tin be described with the states of layers, weights, gates, and candidate layer. Let us assume at time t sec, the memory cell takes \(x_t\), \(H_{t-1}=\) hidden layer output at \(t-1\) sec, \(C_{t-1} =\) memory land of hidden layer at \(t-ane\) sec as input. Every bit output, information technology provides \(H_t\) and \(C_t\).
Gated Recurrent Unit (GRU)
GRU architecture can be distinguished from LSTM past the number of gating units. GRU has a gating unit that determines when to update and what to forget [35]. In the architecture of GRU RNN, there are four main components. It is observed that \(x_t\), \(H_{t-1}\) is provided as input. According to the author of [33], the iv components and their equations are at fourth dimension t
$$\begin{aligned} \text{Update Gate},\quad Z_t\,=\, & {} \sigma (W_zx_t+U_ZH_{t-i}),\end{aligned}$$
(11)
$$\begin{aligned} \text{Reset Gate},\quad R_t\,=\, & {} \sigma (W_r X_t+U_rH_{t-1}),\end{aligned}$$
(12)
$$\begin{aligned} \text{New Memory},\quad H'_t\,=\, & {} \tanh (R_t*UH_{t-1}+Wx_t),\end{aligned}$$
(thirteen)
$$\begin{aligned} \text{Hidden Layer},\quad H_t\,=\, & {} ((1-Z_t )*H'_t)+(Z_t *H_{t-1}). \end{aligned}$$
(xiv)
From the above equations, nosotros see, the current input and previous hidden layers data are reflected in the latest layers. We volition propose a GRU model in the experimental setup affiliate relevant to the above-denoted equations.
Activation Functions
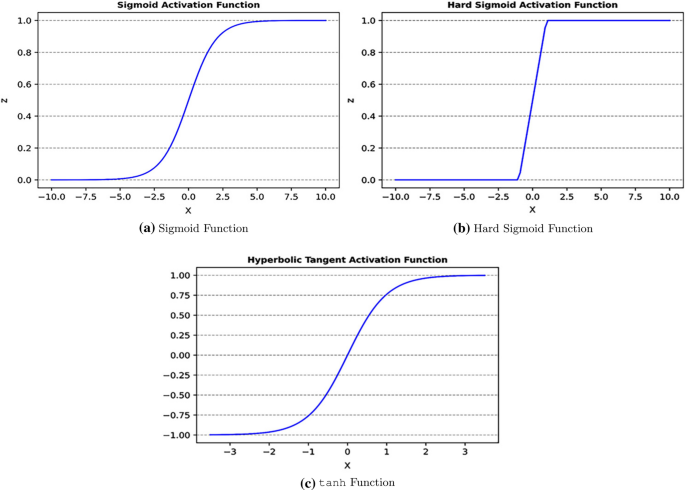
Activation functions
In our model, we used activation function denoted every bit \(\sigma :{\mathrm{I\!R}}\rightarrow {\mathrm{I\!R}}\) which is sigmoid role of Fig. 5a. Sigmoid role takes the sum of weighted inputs and bias equally input, and gives output every bit 0 and 1. The role looks like
$$\begin{aligned} \sigma (z)= \frac{1}{i+eastward^{-z}}. \end{aligned}$$
(fifteen)
For difficult sigmoid, nosotros announce as
$$\begin{aligned} \sigma (z) = \text{max}(0, \text{min}(1,(z+1)/two)). \end{aligned}$$
(xvi)
The hard sigmoid of Fig. 5b is used for the recurrent activation role of LSTM and GRU model instead of soft version, since hard sigmoid produces less computational complexity [36].
The model too uses \(\tanh\) as the activation function as shown in Fig. 5c. The hyperbolic tangent function can be denoted equally
$$\begin{aligned} \sigma (z)=\frac{{\mathrm{e}}^z-{\mathrm{e}}^{-z}}{{\mathrm{e}}^z+{\mathrm{e}}^{-z}}. \stop{aligned}$$
(17)
Automobile Learning Classifier
Popular Python scikit-learn library is imported to implement the following car learning models.
SVM: In SVM, the data points are linearly separated into 2 vector classes by a hyperplane. The credible goal of SVM is to find the hyperplane where margins are the furthest apart. Samples that define the margins are called back up vectors.
Since our dataset is a binary classification problem, the set of possible values that the model can predict is also binary. In the practical example, there are '0' and 'ane', i.e., the dataset label {'Attack': 'one', 'Normal': '0'}, implies that there would be positive support vectors and negative back up vectors nosotros denote them by \(\{+ane, -1\}\). We consider the predictor of the grade
$$\brainstorm{aligned} f: {\mathrm{I\!R}}^D\rightarrow {\{+one,-1\}}. \stop{aligned}$$
(18)
Now, permit u.s.a. derive the hyperplane and the margin [37, 38]. Let 10 be an feature of data space \({\mathrm{I\!R}}^D\) where \({\mathrm{I\!R}}^D \subseteq {\mathrm{I\!R}}\)
$$\begin{aligned} \therefore 10 \mapsto f(ten) = <\varphi ,10> + c ,\quad \text{where}\ \varphi \in {\mathrm{I\!R}}^D \ \text{and} \ c\in {\mathrm{I\!R}}. \end{aligned}$$
(nineteen)
In a general sense, the hyperplane contains no support vectors, i.due east., the distance between \(+i\) elements and \(-ane\) elements would be nada on the hyperplane. When Eq. 19 is nothing, it falls on hyperplane, and therefore if Eq. 19 is greater than or equal to nada, the result would be \(+1\) otherwise \(-one.\) Thus, mathematically, Eq. 19 can be written as follows:
$$\begin{aligned} y_{{\mathrm{prediction}}} = \left\{ \begin{assortment}{ll} -i, \quad \text{if}\ f(x_{{\mathrm{examination}}})<0 \\ +1, \quad \text{if} \ f(x_{{\mathrm{exam}}})\ge 0, \end{array} \correct. \stop{aligned}$$
(20)
where \(y_{{\mathrm{prediction}}}\) is predicted label because \(x_{{\mathrm{test}}}\) as input instances. Separating hyperplane can be defined using kernel tricks. Kernel tin be introduced as similarity function [37] between a pair of instances say \(x_i\) and \(x_j\). For our work, we have followed radial basis function (rbf) kernel, Gaussian kernel is equally follows:
$$\begin{aligned} k(x_i,y_j )= {\mathrm{e}}^{-\Big (\frac{\Vert x_i-y_j\Vert ^2}{two\sigma ^2}\Big )}, \end{aligned}$$
(21)
where \(\Vert x_i-y_j\Vert\) implies Euclidean Norm between two points. The resulting rbf score will autumn into range of \(\{-one, +1\}\).
Boosting: XGBoost is a boosting learning algorithm as well known as Extreme Slope Boosting, implemented in this work. In that location is a divergence between Ensemble Random Wood and XGBoost. Random Forest works equally bagging and aggregation [39]. In dissimilarity, boosting learns from the errors of the previous tree calculating residual errors.
XGBoost can transform a weak predictor into a stronger predictor by summing upward the remainder errors of all trees. With some mathematical indications, we would conclude this section. The loss function for XGBoost [39] is denoted by
$$\brainstorm{aligned} l(\delta )= \Sigma _{i=i}^nl(y_i,{\chapeau{y}}_i^{t-1}+f_t(x_i)), \end{aligned}$$
(22)
where \(l(\delta )\) = loss in mean squared error is computed for tth tree, and \({\hat{y}}\) is the predicted label.
Another distinguishing cistron of XGBoost and ensemble trees is the usage of penalty regularization in XGBoost. The regularization function is equally
$$\begin{aligned} \Psi (\delta )= \gamma L+0.v\beta \Sigma _{i=one}^L\varphi _i^2, \end{aligned}$$
(23)
where \(\gamma\) , \(\beta\) are penalty constants to reduce overfitting, L = number of leaves, and \(\varphi\)= vector space of leaves. Therefore, from Eqs. 22 and 23, the objective function of XGBoost is denoted as-
$$\begin{aligned} \text{obj}(\delta )=l(\delta )+\Psi (\delta ). \terminate{aligned}$$
(24)
Decision Tree: The decision tree supervised learning algorithm is question answer-based model, merely put separate and conquer approach. Question answering model is nothing but tree model, where dataset breaks into several subsets. At the end, information technology contains leafage node which classifies 0 or 1. Moreover, functionality of decision tree as follows:
-
Dividing the mid-point for each set of consecutive unique responses [40].
-
Scoring Criteria to evaluate decision tree partitions such as Entropy and Gini Impurity [vi].
-
Stopping dominion to prevent overfitting problem.
For this experiment, Gini Impurity is considered, based on that decision tree creates a set of rules because training dataset. If there is any examination input or new input is given, the model volition predict the label according to the rule base. Finally, we obtain a tree, where concluding outcome is the leaf node classifies the decision every bit '0' or '1'.
Ensemble Random Forest:To improve the performance and remove a classifier's drawback, developers and scientists usually wait for the ensemble method. Random Forest is a Bootstrap aggregation and bagging ensemble method. Ensemble machine learning is a technique that groups several base models to build a powerful prediction model. For our work, to implement the random forest algorithm python sklearn library is used, sklearn implements the algorithm using Gini Impurity on binary tree [41, 42], and provide output with probabilistic prediction. Therefore, the steps of Random Forest is as follows:
- 1.
Randomly choosing a sample randomly from the dataset with Grand instances and D features.
- ii.
Subset of D features is selected randomly and finding the all-time splitter to split the node iteratively.
- 3.
Growing the tree, and the above steps are repeated until the prediction is given based on the Random Forest characteristic importance formula.
KNN: KNN is a distance-based classifier, and information technology classifies samples based on the closest training examples in the data space. KNN is called lazy learning considering it does not learn discriminative functions. Rather it stores all the grooming data. Lazy learning is case-based learning, with no cost during the training process [43]. The KNN algorithm is straightforward and tin can be summarized every bit
-
Choose K and a distance metric
-
Find theGrand nearest neighbors of a sample that we desire to classify in training information.
-
Based on the larger number of a class, assign the label.
Therefore, the predictor equation of the KNN Classifier [44] can exist denoted as-
$$\begin{aligned} y_{{\mathrm{prediction}}}=\frac{1}{k}\Sigma _{i=1}^Ky_i. \end{aligned}$$
(25)
There are several distance metrics for finding the closest point. Euclidean, Cityblock, Chebyshev, and Minkowski are mostly famous distance metrics.
Experimental Setup
In this department, we describe the settings of our experiment. We illustrate how the class imbalance problem is handled and demonstrate the proposed neural network compages.
Dataset Splitting
We have performed preprocessing, exploratory data analysis, and feature choice on the dataset. After feature selection, we have rechecked for the duplicate. Since some of the features accept been removed, it is no wonder to find a minor number of indistinguishable values. Thus, we removed the copies from the dataset. We have chosen a sample from the population data. Nosotros divided the dataset into ten equal shapes, depending on df['characterization'].value_counts() of data, we chose a chunk to perform the experiment where '0' is the maximum amongst x fragments. Then, the dataset is partitioned into train, examination, and validation datasets. Showtime, the sampled data are split upward with 70% train data and 30% test data. Then, the test data are farther divided into two parts—test set up and validation set with a 50% ratio.
Handling Grade Imbalance Trouble
To solve the class imbalance problem, we usually use the undersampling and oversampling technique. The undersampling approach reduces actual data size; thus, we lost a lot of data. TOMEK is i of the popular under-sampling algorithms [45]. On the contrary, oversampling creates more instances which may atomic number 82 to overfitting; SMOTE performs significantly in oversampling [45]. For our imbalanced dataset, nosotros are applying the SMOTETomek resampling algorithm [46], which combines the under sampling and oversampling technique to reduce the disadvantage of undersampling and oversampling. Using the Python library, the algorithm is implemented to resample our dataset.
Proposed Neural Network Models
After completing initial computations like dataset preprocessing, feature selections, and grade imbalance handling with the appropriate method, the models are trained with the training dataset. Then, the test dataset is fed to predict the built model. In this segment, we will present the proposed model architecture to develop an intrusion detection system.
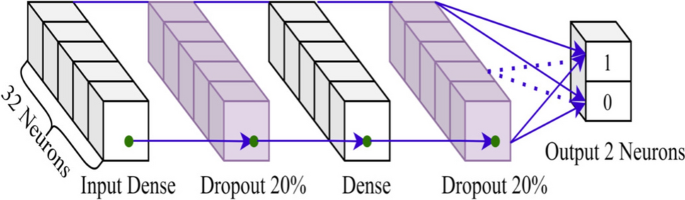
Proposed FFNN architecture
As shown in Fig. 6, there are five layers, one input layer as dense, and the output layer as dense_2. At that place is a dropout layer betwixt two dumbo layers. The model has 32 nodes at each layer except the output layer. The dropout value is twenty% for the proposed model. We recall Eqs. 1–iv to depict the figure. First, the model receives input instances as batch and passes the instances to the kickoff hidden dense layer like to the Eq. 1. Second, the instances propagate through the neurons of dropout layer before feeding into the second dense layer. Finally, the model predicts 'Attack' or 'Normal' form in output layer as the Eq. 4.

Proposed LSTM and GRU architecture
In Fig. vii, we observe viii layers for both LSTM and GRU architecture. There is batch normalization layer, dumbo layers, input and output layer. The input shape and output shape are identical for both models. To empathize the data flow through the model we have to recall from Eqs. 5 to 10 for LSTM and Eqs. 11–14 for GRU model. The showtime layer is input layers which takes instances every bit input in batch and passes it to the cells of LSTM or GRU layers. The output from previous LSTM or GRU enabled layer propagates through rigorous normalization layer earlier feeding to the next LSTM or GRU layer. The layer detailing has been already described in Sect. 4.three of Proposed Methodological Framework chapter. For the deep learning model, we take implemented FFNN, LSTM, and GRU with the help of tensorflow.keras. The following paragraph details some of the important parameters we have used with deep learning models.
Regularization, Optimizer, and Loss Function The goal of regularizers is to forbid overfitting and underfitting. Choosing an advisable epoch number for NN is difficult since too many epochs may lead to overfitting. In dissimilarity, a lower number of epochs may not give the states good accuracy. Since nosotros await good accurateness without any overfitting, we ascertain early stopping callbacks. And then, the model will train until the accurateness of the train split and validation dissever is satisfactory.
The binary cross-entropy loss function is used in our experiment. The GRU RNN uses the aforementioned loss role, optimizer. We have a binary grade dataset; therefore, we would consider thin chiselled cantankerous-entropy as our loss function. We used Adam optimizer for our model, which keeps a history of the previous gradients. Adam optimizer stores exponentially moving average gradients to take momentum like Stochastic Gradient Descent with momentum and squared gradients to calibration learning rate like RMSprop [47].
Evaluation Metrics
The performance of the experiment is measured using the formulas from Eqs. 26 to 29 [43],
$$\begin{aligned} \text{Accuracy}= \frac{\text{TP}+\text{TN}}{\text{Total observations}}, \terminate{aligned}$$
(26)
$$\begin{aligned} \text{Precision}= \frac{\text{TP}}{\text{TP}+\text{FP}}, \end{aligned}$$
(27)
$$\begin{aligned} \text{Recall}= \frac{\text{TP}}{\text{TP}+\text{FN}}, \end{aligned}$$
(28)
$$\brainstorm{aligned} \text{F1 Score}= 2\times \frac{\text{Precision}\times \text{Recall}}{\text{Precision} + \text{Recall}}, \end{aligned}$$
(29)
where TP, TN, FP, and FN are True Positive, Truthful Negative, False Positive, and Imitation Negative. Accuracy measures the number of right classifications penalized by the number of wrong classifications. Precision assesses the quality of a classifier. Call back measures the detection rate that is accurate classifiers penalized by the missed entries while F1 Score measures model performance considering Call back and Precision.
When the dataset is imbalanced, Matthews Correlation Coefficient (MCC) overcomes the class unbalanced raised accurateness bias issue of the classifier [48]. MCC value close to \(+1\) implies a stiff classifier, and close to \(-1\) defines the worst classifier. MCC tin be denoted equally follows:
$$\begin{aligned} \text{MCC} = \frac{\text{TP}\times \text{TN}-\text{FP}\times \text{FN}}{\sqrt{(\text{TP}+\text{FP})(\text{TP}+\text{FN})(\text{TN}+\text{FP})(\text{TN}+\text{FN})}}. \end{aligned}$$
(30)
MCC measures the quality of the classifiers, which is considered to describe our models. Recall, precision would be fatigued where it is necessary. Accurateness score is considered for comparative study with existing works on the Kyoto dataset.
Results Analysis
The experimental results and comparative study are presented and discussed in the following sub sections.
Functioning Significance on Epoch Number
We describe the validity of the proposed neural network models through required evaluation metrics. A set up of hyperparameters is manually tuned during LSTM and GRU training, where the learning rate and lambda of L2 regularizers are 0.001 and 0.003, respectively. The Tabular array three illustrates the findings of the neural network IDS models. The MCC score is considered for 100 and 50 epochs to demonstrate the quality of neural network IDS models. For 100 epochs, the FFNN presents the MCC score equally 94.forty%, where LSTM and GRU IDS models achieve 93.62 and 93.65%, respectively. In terms of fifty epochs, the FFNN, LSTM, and GRU MCC scores are, respectively, 0.68, 0.54, and 0.21% lower than 100 epoch models. Thus, the models surely possess strong MCC scores.
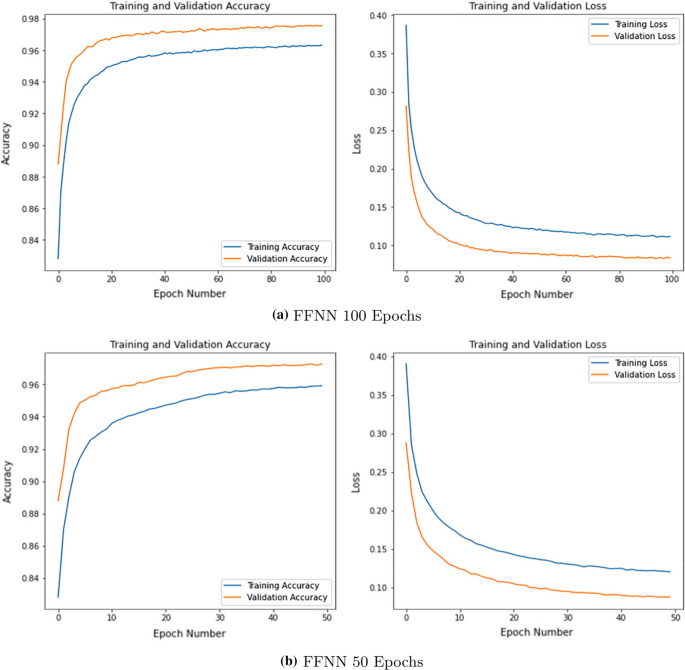
FFNN preparation validation curve for accuracy and loss
Furthermore, from Tabular array 3, the FFNN train and test accuracies are 96.23 and 96.99% for 100 epochs and 95.78 and 96.66% for 50 epochs. For LSTM, the IDS models provide test accuracy of 96.78% for 100 epochs and 96.51% for fifty epochs. The GRU IDS models provide improved performance recording exam accuracies as 96.lxxx and 96.69% for 100 epochs and 50 epochs, respectively. The credibility of such accuracies is further justified by the F1 Score beingness the same for all IDS models 96.61%. The significance of epoch numbers on getting better accuracy tin can be narrated based on the recorded results, leading united states to announce that models are providing meliorate accuracies as the number of epochs is increasing.
Figures 8, ix, and 10 are demonstrated to validate the fitness of the model, which contains Epoch vs Accuracy and Epoch vs Loss curve for 100 epochs and fifty epochs.
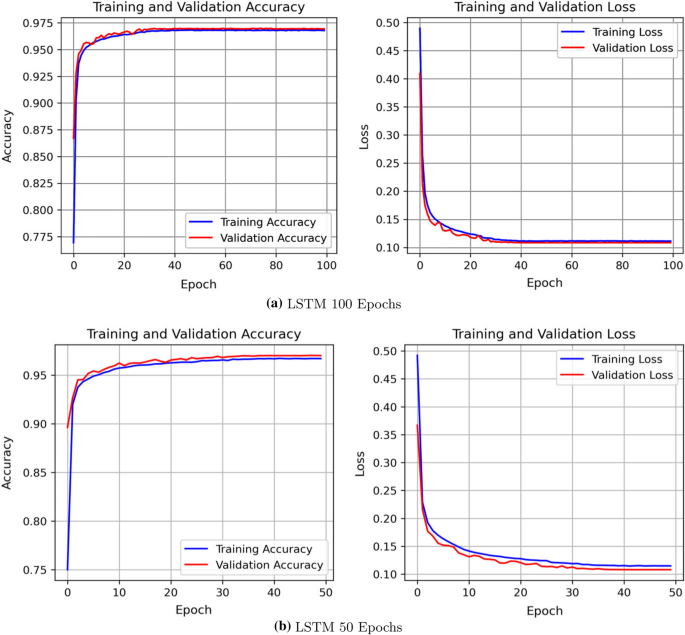
LSTM grooming validation curve for accuracy and loss
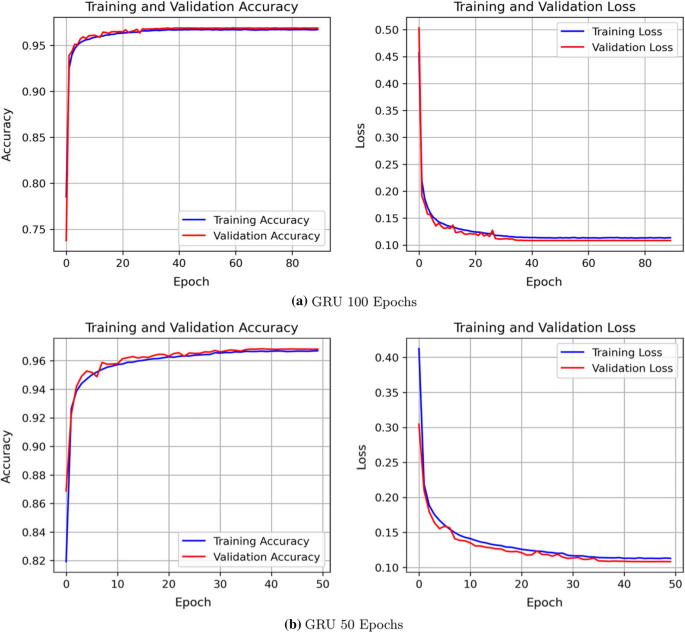
GRU training validation curve for accurateness and loss
These graphs demonstrate no abnormal fluctuation between the training validation curves; the training stops where loss is not entirely 0 either. If the validation loss becomes shut to 0, only then the model becomes overfitting. On the other mitt, the curve is not even underfitting yet since curves demonstrate no overlapping intersection, which somewhen approves perfect fettle.
Early stopping prevents overfitting by keeping an centre on epoch numbers and respective accuracy. For both LSTM and GRU models, the early stopping call back function is provided in the training process. The LSTM of Fig. 9a provides a perfect fit model for 100 epochs, whereas GRU in Fig. 10a performs by preventing overfitting. We observe that the epoch does not reach the 100th epoch due to early stopping; rather, it stops at the 90th epoch. Now discover the accuracy and validation bend for 50 epochs of Figs. 8b, 9b, and 10b. There is satisfactory accuracy and loss, and corresponding plots approve the perfect fitness of the model.
We tin detect that recall and precision of neural network models is identical. The recall is the portion of correct intrusion detection among the existing true positives and faux negatives. The recall implies that models correctly observe the intrusion at a charge per unit of 97% among the existing accurate class. The precision of our proposed neural network models suggests that 97% times the predicted grade is relevant apropos the bodily class.
Machine Learning Model Performances
SVM rbf: From Table 4, the MCC score is 93.89%, defining the quality of the model. The test accuracy is 96.89%, with the predictive power of 95.86%. The accuracy and F1 Score are farther verified with the precision, which is 0.91% college than the retrieve of the model.
Decision Tree: For the decision tree classifier, 98.47% times the classifier detects the actual grade apropos true positives and fake negatives. The precision of 99.70% with 99.10% accuracy justifies the quality of the model. The 99.14% F1 Score is also pregnant. The classifier is potent enough, holding the MCC score is 98.22%. In the Fig. 11, nosotros present the decision tree levels generated from our experiment.
Ensemble Random Woods: In Fig. 12, it is a three-level 20th decision tree of ensemble random forest IDS. The random forest classifier provides 99.17% accuracy with significantly high remember and precision. The predictive power of the model is 99.17% with a significant MCC score of 0.9833.
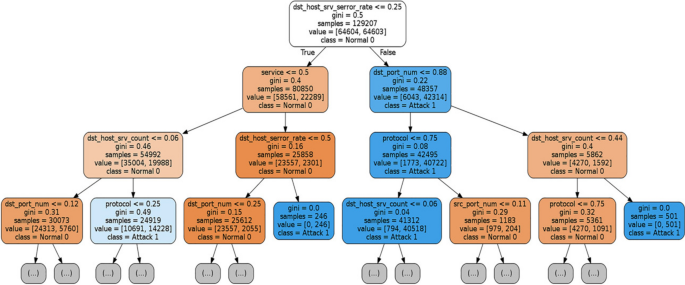
Three-level decision tree
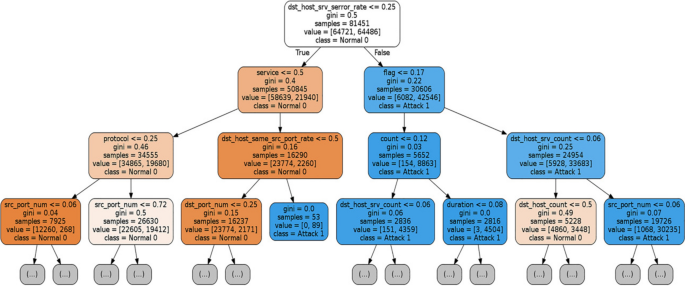
20th three-level decision tree of random woods classifier
KNN: Nosotros have considered G = 5 through manual tuning for the KNN classifier. Nosotros got 98.11% accurateness with a precision of 99.lxxx% and call up is 3.44% lesser than precision. The MCC score is 0.9628, which is satisfactory with the predictive power of 97.44%.
Boosting: We have implemented the XGBoost classifier along with optimization with the assistance of RandomizedSearchCV. The all-time hyperparameters are estimated using 5 cross validation and 25 fitting. XGBoost optimum hyperparameters are: 'min_child_weight': 3, 'max_depth': 15, 'colsample_bytree': 0.five, 'learning_rate': 0.25, and 'gamma': 0.2. XGBoost classifier has generated pregnant accuracy, recall, and precision. The F1 Score defining the predictive power of the model is recorded as 98.79%. The MCC score is very close to +1, which implies it as a strong classifier.
Performance Assessment
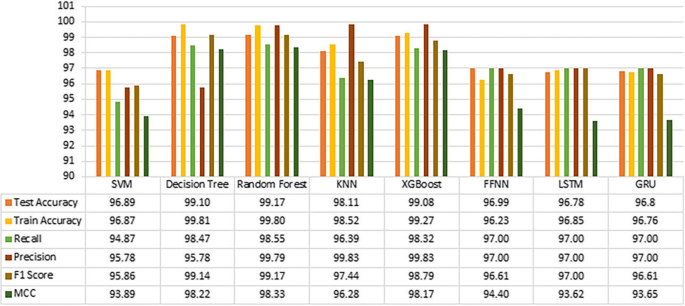
ML and NN IDS model operation comparison
Nosotros are considering 100 epochs neural network IDS models for the assessment since these models are performing better than 50 epochs. As shown in Fig. thirteen, the decision tree works perfectly with the dataset, and random forest performs significantly as well. The KNN algorithm runs a chip slower but faster than SVM producing a meaning MCC Score. In terms of the XGBoost classifier, the MCC score is verifying information technology as a robust classifier. Nosotros can evaluate the Random Forest as the best IDS organization providing 98.33% among the ML models. FFNN neural network model is providing higher accurateness than LSTM and GRU. Based on the MCC score, nosotros must say that all models are performing significantly.
Evaluation with Existing Works
We have developed three traditional machine learning classifiers, two state-of-the-art auto learning models, and 3 deep learning models. It is high time to evaluate how the models are performing with respect to the existing works. Like models of other existing works are considered for this evaluation as shown in Table five.
Zaman et al. [19] denoted the accuracies of 94.26, 97.56, and 96.72% for SVM, KNN, and Ensemble of six models, respectively.
In another piece of work, Vinayakumar et al. [21] implemented a neural network model on Kyoto 2015 dataset and found the accuracy of 88.15%. The authors listed the accurateness of KNN, SVM rbf, Random Forest, and Decision Tree as 85.61, 89.l, 88.20, and 96.xxx%, respectively.
Agarap [20] experimented on the Kyoto 2013 dataset with GRU Softmax and GRU SVM model. The writer listed the accuracies as 70.75% for GRU Softmax, and 84.15% for GRU SVM.
The performances of our proposed neural network models are significant in comparison with existing works. We have improved the accuracies of the neural network model compared with the existing neural network models. In terms of motorcar learning models, our proposed methods are more effective than the listed works in Tabular array 5. Our best accuracy is 99.17% for Random Forest, and the lowest accuracy is 96.36% for SVM rbf, which is also greater than other listed SVM rbf models. The model with GRU Softmax provided less accuracy than GRU Sigmoid, and considering softmax is best fit for multinomial nomenclature [20]. Based on our experimental assay, we announce that for binary classification GRU Sigmoid performs improve than Softmax activated GRU.
Car Learning Black Box Interpretation with LIME
Nosotros take imported LIME to notice what is inside the machine learning black box. In this department, we briefly introduce LIME, and afterwards on, we implement the package with one of our models.
LIME-Explainable AI
Usually, we present the model outcome with some graphical visualizations and tables to explain what we have done. In that location should be a proper authentication procedure for the model so that stakeholders can trust the model to deploy in a particular system. Therefore, LIME takes the responsibleness to testify the trustworthiness and reliability of the model [49]. LIME stands for Local Interpretable Model-agnostic Explanations. LIME helps explain a model whose inner logic is obtuse; in a sentence, LIME explains a machine learning model black box.
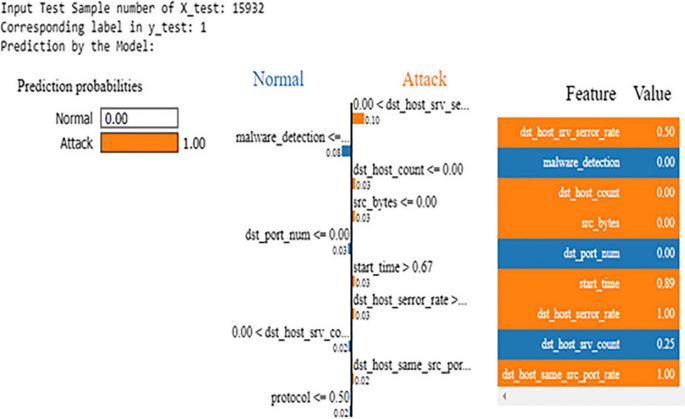
Lime estimation of an instance
The authors of [fifty] discussed the functionality of LIME in detail. The excerptions are as follows: (1) Selecting instances for which explanation of blackness box prediction will be demonstrated. In our example, we used lime_tabular class which takes the preparation dataset as input, (2) The grooming dataset is converted to np.array to get the black box prediction for new samples, (3) LIME uses Gaussian Kernel to give weights to the new points. LIME creates new points from multivariate distribution of features, (four) LIME assigns weights, depending on the proximity between the newly generated points and the data to be explained, (five) The weighted dataset is and then trained using interpretable model, (6) Based on the interpretable model, we obtain the caption of the prediction made on the test instances. Nosotros have implemented the LIME to validate one of the proposed machine learning models.
LIME Implementation
Here, we are because Conclusion Tree as our model to be used as a predictor through LIME interpreter. Nosotros provide prediction function as predict_fn = decisionTree.predict_proba.
Here, nosotros provide input of ten random instances from the test dataset every bit X_test.iloc[g], the LIME explainer displays the explanation with the predicted label for the respective input instance as shown in Fig. 14. For X_test.iloc[23898], the corresponding label is y_test.iloc[23898] is i, and the LIME prediction is 'Set on'. In Tabular array 6, the bodily label and LIME interpretation of the Decision Tree has been reported.
Information technology is proven that our experimented model is working flawlessly to observe the Attack and the Normal intrusion. Thus, if anyone uses this model and gives the input, it would predict accordingly. Let us consider KNN or SVM instead of tree-based classifier or boosting classifier. It volition provide the probabilistic caption in LIME either 56% to Set on or 44% to Normal. Hence, information technology volition detect the input as Attack. Therefore, this is how the machine learning algorithm functions in our network intrusion detection model and assures the reliability of network intrusion detection.
Conclusions
A deep learning-based method is presented in this paper to improve the operation of network intrusion detection. The machine learning models experimented here achieve a considerable improved performance than the peers. Boosting technique is added to SVM, KNN, and DT to conduct the experiment. XGBoost model provides better accuracy than that of the compared models. On the other manus, Ensemble Random Forest achieves the best accuracy, precision, and recall values compared to other models. In addition, both the neural network models, LSTM and GRU, also achieve improved performance while comparing with the country of the arts. However, FFNN shows the all-time performance among all iii deep neural network models. Matthews Correlation Coefficient (MCC) is used to qualify our results, and all the MCC values to close to one, indicating the higher quality of the experimental results. LIME is also used to make the work explainable. Due to the lack of a high configured calculating facility, the experiments are conducted using a role of the dataset. It is our plan to take to the whole dataset for the experiment to get deeper insight of the problem. In addition, as in [12] the experiment can be conducted on other datasets that will permit the states to compare the effectiveness of the proposed method on various datasets. Boosting techniques have been practical in this newspaper; yet, optimization technique, such every bit PSO-based voting ensemble, tin likewise be applied.
References
-
Talele, Northward., Teutsch, J., Jaeger, T., Erbacher, R.F.: Using security policies to automate placement of network intrusion prevention. In: Jürjens, J., Livshits, B., Scandariato, R. (eds.) Engineering Secure Software and Systems, pp. 17–32. Springer, Berlin (2013). https://doi.org/x.1007/978-3-642-36563-8_2
-
Bul'ajoul, West., James, A., Pannu, Thousand.: Improving network intrusion detection system performance through quality of service configuration and parallel engineering science. J. Comput. Syst. Sci. Spec. Issue Optim. Secur. Priv. Trust Eastward-Charabanc. Syst. 81(half dozen), 981–999 (2015). https://doi.org/10.1016/j.jcss.2014.12.012
-
Wang, W., Liu, J., Pitsilis, G., Zhang, X.: Abstracting massive data for lightweight intrusion detection in computer networks. Inf. Sci. 433–434, 417–430 (2018). https://doi.org/ten.1016/j.ins.2016.10.023
-
Belavagi, Thou.C., Muniyal, B.: Performance evaluation of supervised machine learning algorithms for intrusion detection. Procedia Comput. Sci. 89, 117–123 (2016). https://doi.org/10.1016/j.procs.2016.06.016
-
Powers, S.T., He, J.: A hybrid artificial immune system and Self Organising Map for network intrusion detection. Inf. Sci. Nat. Inspir Probl. Solving 178(xv), 3024–3042 (2008). https://doi.org/10.1016/j.ins.2007.eleven.028
-
Suman, C., Tripathy, Due south., Saha, South.: Building an effective intrusion detection system using unsupervised feature pick in multi-objective optimization framework. (2019) CoRR. http://arxiv.org/abs/1905.06562
-
Purwins, H., Li, B., Virtanen, T., Schlüter, J., Chang, S.-Y., Sainath, T.: Deep learning for sound point processing. IEEE J. Sel. Top. Signal Process. 13(2), 206–219 (2019). https://doi.org/10.1109/JSTSP.2019.2908700
-
Gal, Y., Islam, R., Ghahramani, Z.: Deep Bayesian active learning with image data. In: Proceedings of the 34th International Conference on Machine Learning, vol. 70, pp. 1183–1192. PMLR (2017). https://doi.org/x.5555/3305381.3305504
-
Deng, L., Li, J., Huang, J.-T., Yao, K., Yu, D., Seide, F., Seltzer, Thousand., Zweig, G., He, X., Williams, J., et al.: Recent advances in deep learning for speech enquiry at microsoft. In: 2013 IEEE International Conference on Acoustics, Speech and Signal Processing (ICASSP), pp. 8604–8608. IEEE (2013). https://www.microsoft.com/en-united states of america/research/publication/recent-advances-in-deep-learning-for-speech-enquiry-at-microsoft/
-
Rhodes, B.C., Mahaffey, J.A., Cannady, J.D.: Multiple self-organizing maps for intrusion detection. In: Proceedings of the 23rd National Information Systems Security Conference, pp. 16–nineteen. Md Press (2000). https://csrc.nist.rip/nissc/2000/proceedings/papers/045.pdf
-
Fisch, D., Hofmann, A., Ill, B.: On the versatility of radial footing function neural networks: a case written report in the field of intrusion detection. Inf. Sci. 180(12), 2421–2439 (2010). https://doi.org/x.1016/j.ins.2010.02.023
-
Band, Thousand., Wunderlich, S., Scheuring, D., Landes, D., Hotho, A.: A survey of network-based intrusion detection data sets. Comput. Secur. 86, 147–167 (2019). https://doi.org/ten.1016/j.cose.2019.06.005
-
Tsai, C.-F., Hsu, Y.-F., Lin, C.-Y., Lin, W.-Y.: Intrusion detection by car learning: a review. Practiced Syst. Appl. 36(ten), 11994–12000 (2009). https://doi.org/10.1016/j.eswa.2009.05.029
-
Emura, T., Matsui, S., Chen, H.-Y.: compound.Cox: univariate feature selection and compound covariate for predicting survival. Comput. Methods Programs Biomed. 168, 21–37 (2019). https://doi.org/10.1016/j.cmpb.2018.10.020
-
Chormunge, S., Jena, Southward.: Correlation based feature selection with clustering for high dimensional data. J. Electr. Syst. Inf. Technol. 5(3), 542–549 (2018). https://doi.org/10.1016/j.jesit.2017.06.004
-
Radovic, M., Ghalwash, M., Filipovic, Northward., Obradovic, Z.: Minimum redundancy maximum relevance feature selection approach for temporal gene expression information. BMC Bioinform. 18(i), 1–14 (2017). https://doi.org/10.1186/s12859-016-1423-9
-
Vocal, J., Takakura, H., Okabe, Y.: Description of Kyoto University benchmark data (2006). http://www.takakura.com/Kyoto_data/BenchmarkData-Clarification-v5.pdf. Accessed 15 Mar 2016
-
Rai, A.: Explainable AI: from black box to glass box. J. Acad. Marker. Sci. 48(1), 137–141 (2020). https://doi.org/ten.1007/s11747-019-00710-5
-
Zaman, M., Lung, C.-H.: Evaluation of machine learning techniques for network intrusion detection. In: NOMS 2018–2018 IEEE/IFIP Network Operations and Management Symposium, pp. 1–5 (2018). https://doi.org/10.1109/NOMS.2018.8406212
-
Agarap, A.F.Grand.: A neural network architecture combining gated recurrent unit of measurement (GRU) and support vector automobile (SVM) for intrusion detection in network traffic data. In: Proceedings of the 2018 10th International Conference on Machine Learning and Computing. ICMLC 2018, pp. 26–30. Association for Computing Machinery, New York (2018). https://doi.org/10.1145/3195106.3195117
-
Vinayakumar, R., Alazab, Chiliad., Soman, Grand.P., Poornachandran, P., Al-Nemrat, A., Venkatraman, S.: Deep learning approach for intelligent intrusion detection system. IEEE Admission seven, 41525–41550 (2019). https://doi.org/10.1109/ACCESS.2019.2895334
-
Javaid, A., Niyaz, Q., Sunday, West., Alam, Thou.: A deep learning approach for network intrusion detection organisation. In: Proceedings of the 9th EAI International Conference on Bio-Inspired Information and Communications Technologies (Formerly BIONETICS). BICT'15, pp. 21–26. ICST (Plant for Computer Sciences, Social-Information science and Telecommunications Engineering science), Brussels (2016). https://doi.org/x.4108/eai.3-12-2015.2262516
-
Almseidin, M., Alzubi, M., Kovacs, S., Alkasassbeh, K.: Evaluation of auto learning algorithms for intrusion detection arrangement. In: 2017 IEEE 15th International Symposium on Intelligent Systems and Informatics (SISY), pp. 000277–000282 (2017). https://doi.org/10.1109/SISY.2017.8080566
-
Costa, K.A.P., Pereira, L.A.M., Nakamura, R.Y.M., Pereira, C.R., Papa, J.P., Xavier Falcão, A.: A nature-inspired approach to speed upwardly optimum-path wood clustering and its awarding to intrusion detection in computer networks. Inf. Sci. Innov. Appl. Artif. Neural Netw. Eng. 294, 95–108 (2015). https://doi.org/10.1016/j.ins.2014.09.025
-
Song, J., Takakura, H., Okabe, Y.: Cooperation of intelligent honeypots to observe unknown malicious codes. In: 2008 WOMBAT Workshop on Data Security Threats Information Collection and Sharing, pp. 31–39 (2008). https://doi.org/ten.1109/WISTDCS.2008.10
-
García, Due south., Luengo, J., Herrera, F.: Data Grooming Basic Models, pp. 39–57. Springer, Cham (2015). https://doi.org/10.1007/978-three-319-10247-4_3
-
Al-Imran, Yard., Rahaman, K.J., Rasel, 1000., Ripon, Due south.H.: An analytical evaluation of a deep learning model to discover network intrusion. In: Chomphuwiset, P., Kim, J., Pawara, P. (eds.) Multi-disciplinary Trends in Bogus Intelligence, pp. 129–140. Springer, Cham (2021). https://doi.org/x.1007/978-iii-030-80253-0_12
-
Liu, Y., Mu, Y., Chen, K., Li, Y., Guo, J.: Daily action feature selection in smart homes based on Pearson correlation coefficient. Neural Process. Lett. 51(2), 1771–1787 (2020). https://doi.org/10.1007/s11063-019-10185-8
-
Hinton, One thousand.E., Srivastava, N., Krizhevsky, A., Sutskever, I., Salakhutdinov, R.: Improving neural networks by preventing co-accommodation of feature detectors (2012). CoRR. arXiv:1207.0580
-
Phaisangittisagul, E.: An analysis of the regularization between L2 and dropout in single hidden layer neural network. In: 2016 7th International Conference on Intelligent Systems, Modelling and Simulation (ISMS), pp. 174–179 (2016). https://doi.org/10.1109/ISMS.2016.14
-
van Laarhoven, T.: L2 regularization versus batch and weight normalization (2017). CoRR. arXiv:1706.05350
-
Dawani, J.: Hands-On Mathematics for Deep Learning. Packt Publishing Ltd., Birmingham. https://www.packtpub.com/product/hands-on-mathematics-for-deep-learning/9781838647292. Accessed 1 Feb 2021
-
Gumaei, A., Hassan, K.M., Alelaiwi, A., Alsalman, H.: A hybrid deep learning model for human activity recognition using multimodal body sensing information. IEEE Access 7, 99152–99160 (2019). https://doi.org/10.1109/ACCESS.2019.2927134
-
Hochreiter, S., Schmidhuber, J.: Long short-term memory. Neural Comput. 9(viii), 1735–1780 (1997). https://doi.org/ten.1162/neco.1997.nine.8.1735
-
Dey, R., Salem, F.M.: Gate-variants of Gated Recurrent Unit (GRU) neural networks. In: 2017 IEEE 60th International Midwest Symposium on Circuits and Systems (MWSCAS), pp. 1597–1600 (2017). https://doi.org/ten.1109/MWSCAS.2017.8053243
-
Courbariaux, M., Bengio, Y., David, J.-P.: BinaryConnect: training deep neural networks with binary weights during propagations. In: Proceedings of the 28th International Conference on Neural Information Processing Systems, vol. two. NIPS'15, pp. 3123–3131. MIT Press, Cambridge (2015). https://doi.org/ten.5555/2969442.2969588
-
Raschka, Southward.: Python Car Learning. Packt Publishing Ltd., Birmingham. https://www.packtpub.com/production/python-machine-learning-tertiary-edition/9781789955750. Accessed 1 Feb 2021
-
Nguyen Duc, H., Kamwa, I., Dessaint, 50.-A., Cao-Duc, H.: A novel arroyo for early detection of impending voltage plummet events based on the support vector automobile. Int. Trans. Electr. Free energy Syst. 27(nine), 2375 (2017). https://doi.org/10.1002/etep.2375
-
Louppe, G.: Understanding random forests: from theory to practice. PhD thesis (2014). https://doi.org/10.13140/2.ane.1570.5928
-
Myles, A.J., Feudale, R.Northward., Liu, Y., Woody, N.A., Brown, S.D.: An introduction to decision tree modeling. J. Chemom. 18(half-dozen), 275–285 (2004). https://doi.org/x.1002/cem.873
-
Pedregosa, F., Varoquaux, Grand., Gramfort, A., Michel, V., Thirion, B., Grisel, O., Blondel, M., Prettenhofer, P., Weiss, R., Dubourg, V., Vanderplas, J., Passos, A., Cournapeau, D., Brucher, G., Perrot, M., Duchesnay, É.: Scikit-learn: machine learning in Python. J. Mach. Learn. Res. 12(85), 2825–2830 (2011). http://jmlr.org/papers/v12/pedregosa11a.html
-
Buitinck, 50., Louppe, Yard., Blondel, M., Pedregosa, F., Mueller, A., Grisel, O., Niculae, V., Prettenhofer, P., Gramfort, A., Grobler, J., Layton, R., Vanderplas, J., Joly, A., Holt, B., Varoquaux, Chiliad.: API design for car learning software: experiences from the scikit-learn project. In: European Briefing on Machine Learning and Principles and Practices of Cognition Discovery in Databases, Prague, Czech Republic (2013). https://hal.inria.fr/hal-00856511
-
Rezvi, M.A., Moontaha, S., Trisha, K.A., Cynthia, Southward.T., Ripon, South.: Data mining arroyo to analyzing intrusion detection of wireless sensor network. Indones. J. Electr. Eng. Comput. Sci. 21(one), 516–523 (2021). https://doi.org/ten.1016/j.ins.2007.11.028
-
Sébastien, L.: Automobile learning: An practical mathematics introduction. Quant. Fin. 20(three), 359–360 (2020). https://doi.org/10.1080/14697688.2020.1725610
-
Thabtah, F., Hammoud, South., Kamalov, F., Gonsalves, A.: Data imbalance in nomenclature: experimental evaluation. Inf. Sci. 513, 429–441 (2020). https://doi.org/10.1016/j.ins.2019.eleven.004
-
Wang, Z., Wu, C., Zheng, Yard., Niu, 10., Wang, X.: SMOTETomek-based resampling for personality recognition. IEEE Access 7, 129678–129689 (2019). https://doi.org/10.1109/ACCESS.2019.2940061
-
Kingma, D.P., Ba, J.: Adam: A method for stochastic optimization. In: Bengio, Y., LeCun, Y. (eds.) 3rd International Conference on Learning Representations, ICLR 2015, San Diego, CA, Usa, May 7–nine, 2015, Briefing Runway Proceedings (2015). arXiv:1412.6980
-
Chicco, D., Jurman, G.: The advantages of the Matthews correlation coefficient (MCC) over F1 score and accurateness in binary classification evaluation. BMC Genom. 21 (2020). https://doi.org/x.1186/s12864-019-6413-seven
-
Ribeiro, M.T., Singh, S., Guestrin, C.: "Why should I trust you?": explaining the predictions of whatever classifier. In: Proceedings of the 22nd ACM SIGKDD International Briefing on Knowledge Discovery and Data Mining. KDD '16, pp. 1135–1144. Association for Computing Machinery, New York (2016). https://doi.org/10.1145/2939672.2939778
-
Visani, Yard., Bagli, E., Chesani, F., Poluzzi, A., Capuzzo, D.: Statistical stability indices for lime: obtaining reliable explanations for auto learning models. J. Oper. Res. Soc. 1–xi (2021). https://doi.org/10.1080/01605682.2020.1865846
Author information
Affiliations
Corresponding writer
Ethics declarations
Conflict of interest
The authors report no conflict of interest. The authors alone are responsible for the content and writing of this article.
Additional information
Publisher'south Note
Springer Nature remains neutral with regard to jurisdictional claims in published maps and institutional affiliations.
Rights and permissions
Open Admission This commodity is licensed under a Artistic Commons Attribution 4.0 International License, which permits employ, sharing, adaptation, distribution and reproduction in any medium or format, every bit long as you lot give advisable credit to the original author(s) and the source, provide a link to the Creative Commons licence, and point if changes were made. The images or other third party material in this article are included in the article's Creative Commons licence, unless indicated otherwise in a credit line to the fabric. If cloth is not included in the article's Creative Commons licence and your intended employ is not permitted by statutory regulation or exceeds the permitted use, you will need to obtain permission directly from the copyright holder. To view a copy of this licence, visit http://creativecommons.org/licenses/by/4.0/.
Reprints and Permissions
About this commodity
Cite this article
Al-Imran, G., Ripon, S.H. Network Intrusion Detection: An Analytical Assessment Using Deep Learning and State-of-the-Art Machine Learning Models. Int J Comput Intell Syst 14, 200 (2021). https://doi.org/10.1007/s44196-021-00047-iv
-
Received:
-
Accepted:
-
Published:
-
DOI : https://doi.org/ten.1007/s44196-021-00047-4
Keywords
- Intrusion detection organisation
- Optimization
- Long short-term memory (LSTM)
- Deep learning
- Form imbalance
- Ensemble method
Source: https://link.springer.com/article/10.1007/s44196-021-00047-4